Adapt or Die
Name a company anywhere in the world – just one! – that won’t be disrupted by Amazon or Google. These two companies, along with their fellow votaries of Big Data and machine learning, are today entering every industry, to the competitive peril of businesses large and small.
The healthcare and financial industries are among those most at risk of disruption from AI. To date, attempts to meet this disruption seem to have ranged from wan incrementalism to the abjectly sclerotic. Truly addressing AI’s disruption will require that all companies become AI-driven disruptors themselves. In business, in the battle between “incremental” and “disruptive”, “incremental” seldom wins. But how exactly should companies not named Amazon or Google build disruptive AI solutions?
Swimming with the Sharks
The best templates for how to build a data-driven business lie in plain view: Amazon, Google, Uber, and a host of lesser-known technology companies whose singular goal is to build disruptive business models exclusively on the foundation of data. The common thread through each of these companies is their near-religious fixation on multi-dimensional (“hyper-dimensional”) data sets, deployed using multi-dimensional methods. Let’s break down what this all means into 7 best practices:
- Start with the business model. Period. If there’s no compelling economic advantage underlying the business model, then no amount of AI or data will make an iota of impact. Amazon and Google both share the common trait of focusing only on applications with quantifiable benefits in multi-billion-dollar markets which support frictionless scaling. And it’s the sheer and enduring size of the “health and wealth” markets that now attracts the baleful gaze of these AI-based disruptors.
- Predict the future. Data can be interpreted in two ways: to gain an understanding of the past, or to gain an understanding of the future. A single-dimensional approach to interpreting data would be to simply do the former: analyze the data – and “Big” it might be – to determine what’s already happened. Interesting business intelligence, but of tactical value in a landscape where every industry has been commoditized. Strategic value in commoditized markets lies in being able to predict the future. It is in this added dimension of data analysis where companies such as Amazon and Google excel.
Within healthcare, for example, there is unquestionable value in the application of machine learning to correctly diagnose a biopsy image. But diagnosis is only a single (retrospective) dimension of a data set’s semantics. Prediction adds multi-dimensional semantics to data, and it is prediction that helps drive prevention (or at a minimum, early intervention), which in turn drives cost reduction. Machine learning applied to predicting the future of health states – in everything from mood and anxiety disorders to cardiovascular mortality and general population health – is how healthcare might emulate what Amazon has already done in the much more prosaic industry of retail logistics. - Data-richness: through proprietary data sources. If data is the ultimate source of value (and it is!), then the defensibility of any technology or business is predicated on identifying a proprietary data source. (See how Cozi has done this). A proprietary data source is merely a starting point, however. If data constitutes the ultimate source of value then even more proprietary data – i.e., data-richness – is always required: data must continually be driven to become more multi-dimensional. Take Google as an example. Even though the company produces data that is already proprietary and massively-dimensional, Google recently spent over $2 billion to acquire Fitbit. Fitbit’s multi-dimensional health data is now part of Google’s proprietary data stream: that’s the religion of data-richness put in practice.
Amazon too is massively-dimensional and focused on data-richness. The company isn’t satisfied simply with capturing your online and offline (Whole Foods!) browsing and purchase data. We know from academic literature that both facial images and voice signals can be correlated to determine the states of human health. It’s then no surprise that Amazon’s relentless pursuit of data-richness has manifested in healthcare patents for both facial and voice analytics. - Data-hungriness: for multi-dimensional data from external sources. If data is good, then more data is better. This includes multi-dimensional data obtained from any and all external sources, whether those sources are freely available (e.g., from public entities) or available through partnerships. The genius in Uber’s 2013 patent, “System and Method for Dynamically Adjusting Prices for Services”, is not some impenetrable machine learning technology but the broad multi-dimensionality of the data streams Uber ingests to accurately predict pricing for a commodity (transportation) service: everything from weather, to voting days or first day of school for a city, to social networking data, to flight information from airports and airlines, to news information, etc. You get the idea.
Writing for the Federal Reserve Bank of Philadelphia Julapa Jagtiani and Catharine Lemieux reported that multi-dimensional (“alternative”) data in fintech lending now enables the provision of “increased access to credit at a lower cost to those creditworthy individuals who have thin credit history”. Fintech start-up Prosper “gets 500 pieces of data on each borrower”, and others such as SoFi and Kabbage have been able to leverage multi-dimensional data to move away from FICO scores altogether to find those well-deserving “invisible prime” customers.
What might some of these deterministic, multi-dimensional data signals be? Tobias Berg, et al., writing for the FDIC reported that, among other things, time of day, whether customers have their names in their email addresses, and whether customers made typing mistakes all correlate to loan risk. Chinese fintech analyzes more than 1,200 data points to determine loan risk for under-banked consumers. This includes mobile phone data to correlate risk to people who don’t answer phone calls or whose outgoing calls go unanswered. - Drive future actions using behavioral economics. Amazon, Google and Uber are passed masters in completing the “cycle” of data using multi-dimensional methods. Specifically, all combine data with behavioral economics to drive end-user behavior in ways advantageous to their businesses. The responses to these behavioral economics methods are constantly monitored and fed back into the machine learning as yet another optimizing multi-dimensional data stream.
In the fintech space, start-ups like Lemonade are using behavioral economics to drive positive user behavior. But behavioral economics as a multi-dimensional method — through gamification, nudging, defaults, the decoy effect — need not be used solely for the purposes of increasing revenue. Behavioral economics have been applied to a range of healthcare applications such as driving colorectal cancer screening, organ donation and smoking cessation, and even to improving heart failure care and surgical hygiene. - Measure the results and refine in real-time. It’s important to realize that the products of the first 5 steps above are a) a revenue stream; AND b) a data stream. The data stream measures and reports on the accuracy of the future-prediction and the effectiveness of the behavioral economics. These learnings constitute yet another multi-dimensional method: they’re fed back into the system so that algorithms, data sources and techniques can be constantly corrected and improved.
- A final multi-dimensional method, and one perhaps not engineered into AI-driven companies founded in recent years, is to build ethics into the business model from Day One. Be ethical. Period. Source the data ethically, and deploy the data ethically. Behaving ethically means not just having a policy in place, but constantly questioning the ethical impact of every decision and action. It means being ethical both when the AI decides for us, and also when we decide based on the AI’s recommendations. It’s a process of constant vigilance. Only through ethics can we build differentiated businesses that can compete and last.
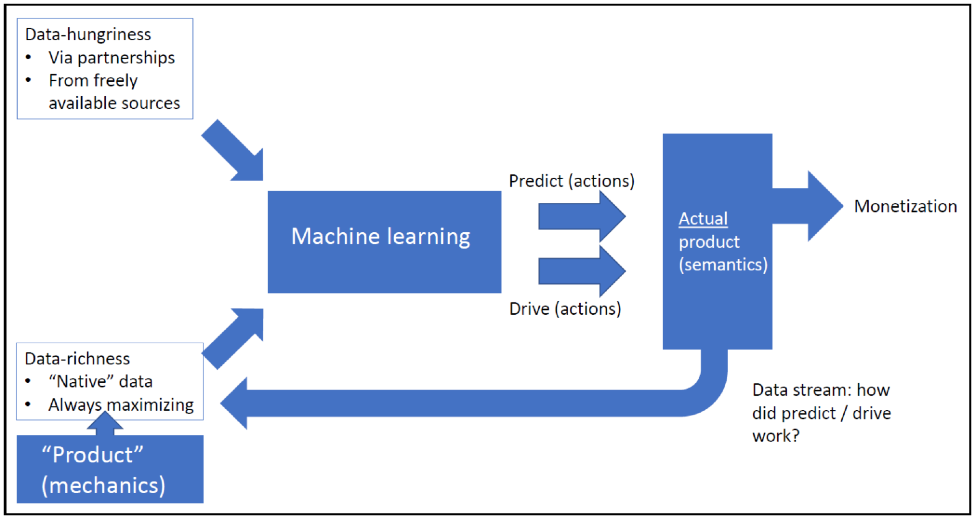
Being Competitive, Being Relevant
Adhering to the best practices above are no guarantee of success, in healthcare, fintech or in any other industry. But bringing these guidelines into discussion might help organizations to, at a minimum, be properly girded for the inevitable combat with the Big Tech practitioners of AI. If your future competitors will wield multi-dimensional data in multi-dimensional ways, you’d best plan for the same while there’s still time. It may be that the specifics of your business model do not require adherence to all seven “habits”. But rule everything in (by default) before you rule anything out (after pained deliberation!). Disrupting your own business is hard. But it’s better to do so yourself than have a competitor do it for you.
Disruption will come to every industry. Done right, this disruption will help bring deserved services to un-served populations. It will also bring scalable (cheaper) services to those already enjoying technology’s benefits, and do so in a manner that is ethical and sustainable. Not doing disruption right – by taking a narrow-dimensional view of innovation — will cede AI-driven disruptions to a monopolistic few companies. The “haves” will have more, and the “have-nots” will have naught.
Adapt or Die
Name a company anywhere in the world – just one! – that won’t be disrupted by Amazon or Google. These two companies, along with their fellow votaries of Big Data and machine learning, are today entering every industry, to the competitive peril of businesses large and small.
The healthcare and financial industries are among those most at risk of disruption from AI. To date, attempts to meet this disruption seem to have ranged from wan incrementalism to the abjectly sclerotic. Truly addressing AI’s disruption will require that all companies become AI-driven disruptors themselves. In business, in the battle between “incremental” and “disruptive”, “incremental” seldom wins. But how exactly should companies not named Amazon or Google build disruptive AI solutions?
Swimming with the Sharks
The best templates for how to build a data-driven business lie in plain view: Amazon, Google, Uber, and a host of lesser-known technology companies whose singular goal is to build disruptive business models exclusively on the foundation of data. The common thread through each of these companies is their near-religious fixation on multi-dimensional (“hyper-dimensional”) data sets, deployed using multi-dimensional methods. Let’s break down what this all means into 7 best practices:
- Start with the business model. Period. If there’s no compelling economic advantage underlying the business model, then no amount of AI or data will make an iota of impact. Amazon and Google both share the common trait of focusing only on applications with quantifiable benefits in multi-billion-dollar markets which support frictionless scaling. And it’s the sheer and enduring size of the “health and wealth” markets that now attracts the baleful gaze of these AI-based disruptors.
- Predict the future. Data can be interpreted in two ways: to gain an understanding of the past, or to gain an understanding of the future. A single-dimensional approach to interpreting data would be to simply do the former: analyze the data – and “Big” it might be – to determine what’s already happened. Interesting business intelligence, but of tactical value in a landscape where every industry has been commoditized. Strategic value in commoditized markets lies in being able to predict the future. It is in this added dimension of data analysis where companies such as Amazon and Google excel.
Within healthcare, for example, there is unquestionable value in the application of machine learning to correctly diagnose a biopsy image. But diagnosis is only a single (retrospective) dimension of a data set’s semantics. Prediction adds multi-dimensional semantics to data, and it is prediction that helps drive prevention (or at a minimum, early intervention), which in turn drives cost reduction. Machine learning applied to predicting the future of health states – in everything from mood and anxiety disorders to cardiovascular mortality and general population health – is how healthcare might emulate what Amazon has already done in the much more prosaic industry of retail logistics. - Data-richness: through proprietary data sources. If data is the ultimate source of value (and it is!), then the defensibility of any technology or business is predicated on identifying a proprietary data source. (See how Cozi has done this). A proprietary data source is merely a starting point, however. If data constitutes the ultimate source of value then even more proprietary data – i.e., data-richness – is always required: data must continually be driven to become more multi-dimensional. Take Google as an example. Even though the company produces data that is already proprietary and massively-dimensional, Google recently spent over $2 billion to acquire Fitbit. Fitbit’s multi-dimensional health data is now part of Google’s proprietary data stream: that’s the religion of data-richness put in practice.
Amazon too is massively-dimensional and focused on data-richness. The company isn’t satisfied simply with capturing your online and offline (Whole Foods!) browsing and purchase data. We know from academic literature that both facial images and voice signals can be correlated to determine the states of human health. It’s then no surprise that Amazon’s relentless pursuit of data-richness has manifested in healthcare patents for both facial and voice analytics. - Data-hungriness: for multi-dimensional data from external sources. If data is good, then more data is better. This includes multi-dimensional data obtained from any and all external sources, whether those sources are freely available (e.g., from public entities) or available through partnerships. The genius in Uber’s 2013 patent, “System and Method for Dynamically Adjusting Prices for Services”, is not some impenetrable machine learning technology but the broad multi-dimensionality of the data streams Uber ingests to accurately predict pricing for a commodity (transportation) service: everything from weather, to voting days or first day of school for a city, to social networking data, to flight information from airports and airlines, to news information, etc. You get the idea.
Writing for the Federal Reserve Bank of Philadelphia Julapa Jagtiani and Catharine Lemieux reported that multi-dimensional (“alternative”) data in fintech lending now enables the provision of “increased access to credit at a lower cost to those creditworthy individuals who have thin credit history”. Fintech start-up Prosper “gets 500 pieces of data on each borrower”, and others such as SoFi and Kabbage have been able to leverage multi-dimensional data to move away from FICO scores altogether to find those well-deserving “invisible prime” customers.
What might some of these deterministic, multi-dimensional data signals be? Tobias Berg, et al., writing for the FDIC reported that, among other things, time of day, whether customers have their names in their email addresses, and whether customers made typing mistakes all correlate to loan risk. Chinese fintech analyzes more than 1,200 data points to determine loan risk for under-banked consumers. This includes mobile phone data to correlate risk to people who don’t answer phone calls or whose outgoing calls go unanswered. - Drive future actions using behavioral economics. Amazon, Google and Uber are passed masters in completing the “cycle” of data using multi-dimensional methods. Specifically, all combine data with behavioral economics to drive end-user behavior in ways advantageous to their businesses. The responses to these behavioral economics methods are constantly monitored and fed back into the machine learning as yet another optimizing multi-dimensional data stream.
In the fintech space, start-ups like Lemonade are using behavioral economics to drive positive user behavior. But behavioral economics as a multi-dimensional method — through gamification, nudging, defaults, the decoy effect — need not be used solely for the purposes of increasing revenue. Behavioral economics have been applied to a range of healthcare applications such as driving colorectal cancer screening, organ donation and smoking cessation, and even to improving heart failure care and surgical hygiene. - Measure the results and refine in real-time. It’s important to realize that the products of the first 5 steps above are a) a revenue stream; AND b) a data stream. The data stream measures and reports on the accuracy of the future-prediction and the effectiveness of the behavioral economics. These learnings constitute yet another multi-dimensional method: they’re fed back into the system so that algorithms, data sources and techniques can be constantly corrected and improved.
- A final multi-dimensional method, and one perhaps not engineered into AI-driven companies founded in recent years, is to build ethics into the business model from Day One. Be ethical. Period. Source the data ethically, and deploy the data ethically. Behaving ethically means not just having a policy in place, but constantly questioning the ethical impact of every decision and action. It means being ethical both when the AI decides for us, and also when we decide based on the AI’s recommendations. It’s a process of constant vigilance. Only through ethics can we build differentiated businesses that can compete and last.
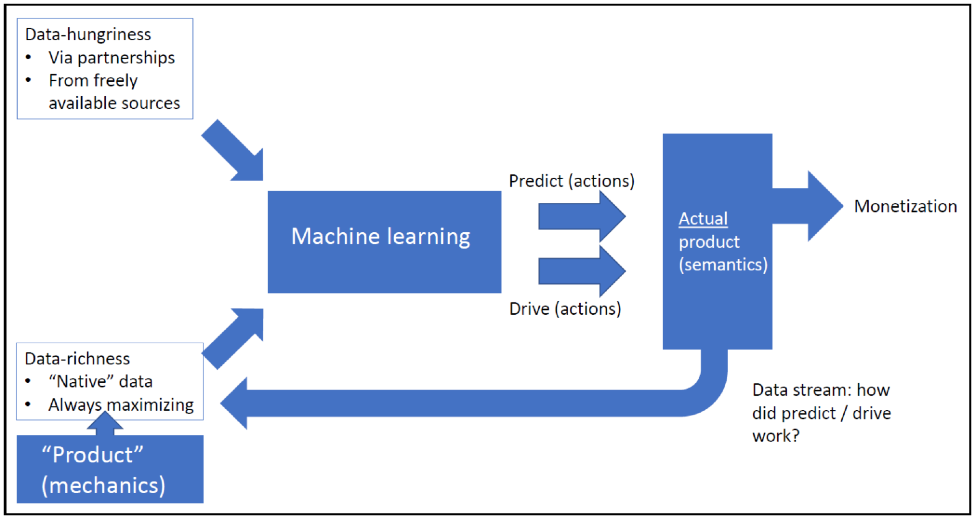
Being Competitive, Being Relevant
Adhering to the best practices above are no guarantee of success, in healthcare, fintech or in any other industry. But bringing these guidelines into discussion might help organizations to, at a minimum, be properly girded for the inevitable combat with the Big Tech practitioners of AI. If your future competitors will wield multi-dimensional data in multi-dimensional ways, you’d best plan for the same while there’s still time. It may be that the specifics of your business model do not require adherence to all seven “habits”. But rule everything in (by default) before you rule anything out (after pained deliberation!). Disrupting your own business is hard. But it’s better to do so yourself than have a competitor do it for you.
Disruption will come to every industry. Done right, this disruption will help bring deserved services to un-served populations. It will also bring scalable (cheaper) services to those already enjoying technology’s benefits, and do so in a manner that is ethical and sustainable. Not doing disruption right – by taking a narrow-dimensional view of innovation — will cede AI-driven disruptions to a monopolistic few companies. The “haves” will have more, and the “have-nots” will have naught.