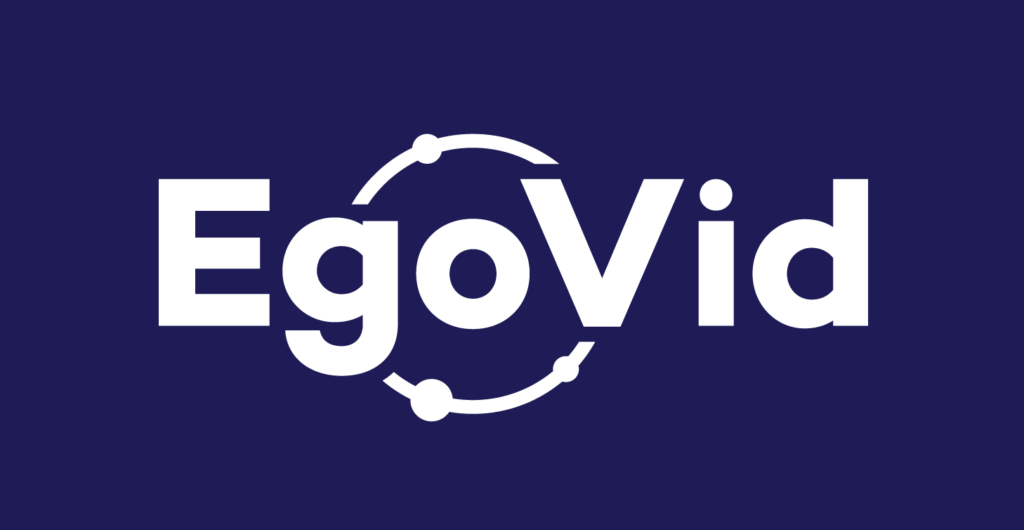
According to IBM, the cognitive computing market size presents a $2 trillion dollar opportunity over the next decade. One of the biggest components of this metric falls into the category of machine learning. While many have been using it as an overarching term to refer to a myriad of technological advancements and applications, visionary entrepreneurs and researchers from EgoVid are focusing their efforts on solving a clearly defined problem they are certain will directly impact the average individual’s willingness to engage in upcoming waves of innovation: how to allow companies to utilize user data to create advanced machine learning algorithms without compromising their privacy or exposing them to cyber threats.
This problem might seem distant at first, but the reality is we just need to look back at the latest release made yesterday by Wikileaks documenting the CIA’s hacking tools to see how user data can be exposed. Or as Mary Dejevsky explains it in her most recent piece, we are entering a time where you might be watching the TV at the same time the TV is watching you back.
In fact, there appears to be no question about whether our privacy has been compromised with the advent of technical disruption.
We had the pleasure of discussing EgoVid and the future of machine learning with CoFounder and CEO Professor Hyun Jong Yang and Machine Learning Researcher Kiyoon Kim at the Sutardja Center who are also affiliated with the Ulsan National Institute of Science and Technology (UNIST), an important Global Partner for the SCET. During our conversation, they shared with us their experience of the past two months since they have been visiting Berkeley, and the ways in which they have been able to strategically position their venture for upcoming challenges and opportunities.
CRV:Can you start by giving us a brief introduction to EgoVid and what is the problem your initiative is focusing on?
HY: We work in machine learning, particularly in “safe” machine learning. Nowadays, the most popular application of machine learning is concerned with improving computer vision. Using machine learning to improve computer vision, you can recognize objects, targets or even situations by just analyzing data inputs such as images or video clips. For example, Google has been great in doing just this by analyzing all of the data collected from individual submissions of users on platforms such as YouTube. However, with all of these data pieces, there is inevitably a privacy issue that eventually arises.
CRV:What do you mean by a privacy issue? Are these companies purposefully violating users’ private space?
KK: Let’s say that you want to be on board with the new technological advancement as it relates to making your home “smarter”. As part of a smart home system, you will have to install several cameras around your house in order for the system to perform even the most simple tasks, such as adapting the lighting automatically when you walk into your dining room. This task can only be performed by a camera or sensor installed in the area that can be exposed to hacking by a third party. When a hacker intervenes with your smart home system a lot of private information pertaining to your personal life could be accessible to the attacker.
CRV: Who are the players who are recognizing this need the most? Is it big data analytics companies? Do you feel the average consumer has enough information about machine learning processes to feel that his personal privacy is in jeopardy?
HY: We definitely see the pressure for adoption of safer systems from the consumer, not the big companies. The average individual is the one who should and will care about big firms having access to high-quality information regarding his private life. People are already aware of this problem. Policeman, for example, already wear a kind of a first-person camera system to recognize, not record, their day to day interactions with civilians. It is similar to a GoPro. There has been significant discomfort from people from an intrusion of privacy perspective coming out of policeman carrying these cameras and filming them at all times.
KK: In the future, we expect an important rise in assistive technology in the form of robots. Robots will be assisting people, and they will need to have cameras incorporated into their systems so they have the ability to “see”. But, what happens if that visibility device is intervened by a third party? People need to be aware of the potential of both disruption and intrusion that will comes from machine learning. This is the reason EgoVid’s goal is to create algorithms that promote the concept of “safe” machine learning applications.
“We know that in the future there are going to be privacy issues with machine learning. We just happen to be ahead of the curve.”
– Hyun Jong Yang, CEO EgoVid.
CRV:Not to play devil’s advocate, but from an implementation standpoint, wouldn’t making algorithms “safer” by restricting the quality of data available to them compromise the accuracy of the algorithm and the entire system?
HY: From a traditional standpoint, you are right. Every time that the quality of the data fed into the algorithms worsens, the accuracy of the algorithm’s output reduces significantly. This is exactly the problem that EgoVid has been working on. We want to maintain the algorithms’ high accuracy without needing high resolution images, video clips, or pieces data.
KK: It is important to note, however, that the output of the algorithm will not carry the same level of accuracy than when using high resolution data, but its accuracy will still be high enough to classify objects from the pieces of data that are being analyzed.
CRV:You have been working on EgoVid since August 2016. In what stage of development is the project right now?
HY: We have written a research paper about the topic and presented it in the AAAI Artificial Intelligence Conference. Our research publication centered primarily around situation recognition using very low resolution video. We were very satisfied with the results that we were able to achieve with our technology. With the video dataset with which we tested our approach, we were able to obtain a 96% situation recognition rate. This being said, our investors want us to sell something and not only keep this as a platform for further research. Commercialization is very important for them.
CRV:Have you identified any key markets that you might be able to disrupt by applying your algorithms?
HY: We have defined CCTV camera surveillance systems as our primary market. Lots of CCTV cameras are already installed, but the image that you get from the video recording is usually in a very low resolution, especially when cameras are strategically hidden far away from individuals’ sight. If you apply our algorithm to this very conventional market you have the potential of increasing the identification rate of the object and people in the video recordings.
KK: The key here is that we will be taking advantage of the fact that all of the cameras that are part of these CCTV systems have already been installed. Our algorithm would simply be dealing with changing their software, providing us with a high degree of scalability. We have also already received a couple of offers from organizations and institutions in Korea that are seeking to implement our algorithm in their systems.
CRV: What motivated you to come to the United States and work in EgoVid? And once you decided to come here, why did you see Berkeley and the SCET as attractive locations to develop the project, and what is your most important takeaway from your visit?
HY: Lots of global companies are interested in computer vision machine learning, including Google, Microsoft and Facebook. Eventually and inevitably, these companies will become our competitors. As a standalone company, we wouldn’t have a problem of surviving in Korea, but when we look at the global landscape, the fundamental question would be whether we could compete with these corporations. For now, our strategic plan is to grow and generate significant revenue in Korea while we improve our algorithms even further. At some point, we are planning to sell EgoVid’s whole intellectual property to a global company. We believe that we will be selling it to one of these big American conglomerates, so our primary incentive to come to the United States was to develop relationships with these potential buyers.
KK: I would say one of our biggest achievements was to bring on board several advisors who will be crucial for our venture’s development both in the near future and in the potential acquisition process in the long run. In terms of our experience with Berkeley and the SCET, besides the rich faculty engagements we have had during the past months, we confirmed that the University was a great place to have settled within the Bay since it is located relatively close to several key places that we needed to travel to gain exposure.